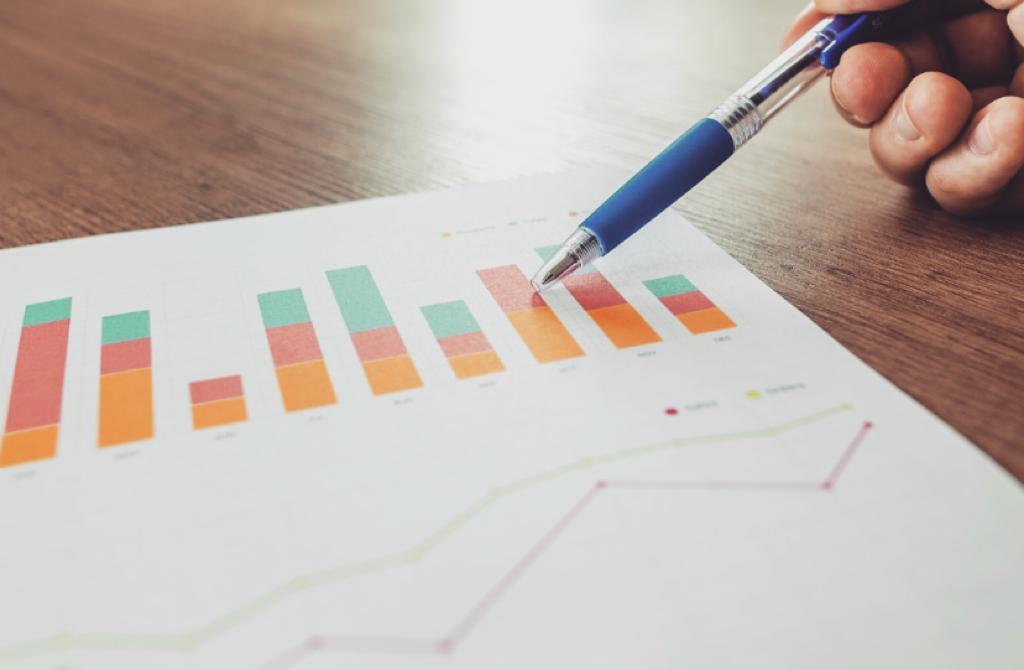
How to Apply Regression Discontinuity to Measure Impact
In many public programs, the decision about who are the beneficiaries of a program—and who aren’t—is made based on some ranking or continuous variable, e.g. a test score or a household’s income level. In these cases, randomization to measure the impact of the program is unfeasible, and analysts must resort to quasi-experimental techniques.
Ana, a 17-year-old student from Colombia, has just finished the 11th grade. She lives in a low-income household where education is a great value. This year she will take the SABER 11 standardized test and hopes to earn a grade above 342; if she does, she will be able to access higher education scholarships offered by the Colombian government.
Ricardo and Adriana are a couple and have a baby. They are planning to stop renting and buy a home in Argentina. Between the two, the household income equals 4 minimum wages. They have some savings and need a mortgage loan to cover part of the property price. They will apply to become beneficiaries of the ProCreAr program. If their score—calculated based on household data—is above the cut-off point, they will be eligible for a loan plus a government subsidy.
In many public programs, the decision about who are the beneficiaries of a program—and who aren’t—is made based on some ranking or continuous variable, e.g. a test score or a household’s income level. In these cases, randomization to measure the impact of the program is unfeasible, and analysts must resort to quasi-experimental techniques.
When access to a program’s benefits is possible only for those with a score over a given threshold, the go-to impact assessment methodology is Regression Discontinuity (RD).
RD is divided into 4 components:
- Each analysis unit has a score: in our examples, these would be the scores on the SABER 11 test and the score to access housing credit.
- An objective assignment rule (one that cannot be manipulated or predicted by applicants). For example, only applicants with scores higher than 342 will receive the scholarship.
- The cut-off point or threshold: a score of 342 on the SABER 11 test.
- The intervention: the granting of the credit for recipients of the Ser Pilo Paga (SPP) program and the granting of mortgage loans and subsidies to ProCreAr beneficiaries.
In addition, in order to implement the RD design, 3 requirements must be met:
- The behavior of non-treatment characteristics should be continuous around the cut-off point. Analysts must confirm that the only value that changes around the cut-off point is the probability of treatment. The other dimensions that characterize the students who took the SABER 11 test and the households that applied to the ProCreAr program behave continuously. In other words, the average age of those who scored 342 or higher should not be different from those who scored less than 342; and the average income of households that scored higher than the ProCreAr cut-off point should not differ from those with scores below that threshold. If that were the case, then changes in granting the scholarships, or the change of access to mortgage credits (and subsidies) could not be objectively attributed, because the scholarship, or the credit-subsidy, would not be the only changing variable between the treatment and the control groups.
- There should be no manipulation in treatment assignment around the cut-off point. It should be established that the factor that determines who receives the scholarship (or credit-subsidy) and who does not, is only the score, higher than 342 (ProCreAr threshold), and not willful attempts of students (or families) to manipulate the assignment rule or move their score above or below the cut-off point. If there is any irregularity in the treatment assignment, those around the cut-off point will not necessarily be similar, which would prevent us from attributing causality to scholarships (or subsidies-credits) in case assignment (access to housing) is different between the two groups.
- The likelihood of treatment increases drastically at the cut-off point. The likelihood of receiving the scholarship (or credit-subsidy) significantly increases at 342 or a higher score (ProCreAr cut-off point).
Finally, once these conditions have been verified, we will want to measure the impact of these programs. To this end, we will require:
- to define result variables: We will be interested to know the impact of the SPP program on the enrolment rate in universities, while in the case of ProCreAr beneficiaries we will be able to measure the average difference in access to credits across the control and treatment groups.
- to define observation weighting: In general, more weighting is usually assigned to observations closest to the cut-off point, since those farther on either side the threshold tend to be more similar.
- to select the bandwidth: to select which score (above and below the cut-off point) will be used to measure impact. It should be noted that there is a bias-variability “trade-off”: the wider the better because we will use more observations. However, the impact estimation will have a lower quality because the groups will not be as similar to each other.
- Lastly, in simplified terms, estimating the impact with an RD design is equivalent to establishing a mean variation in the results of the variable of interest for observations above and below the cut-off point. In more sophisticated terms, this involves performing two regressions and calculating the difference of the coefficients that accompany the impact variable in question.
If you are interested in learning more about this methodology, theory and applications, as well as other quasi-experimental impact measurement methodologies, you can sign up for our intermediate MOOC that will be available from August on our microsite.
CAF's more recent content
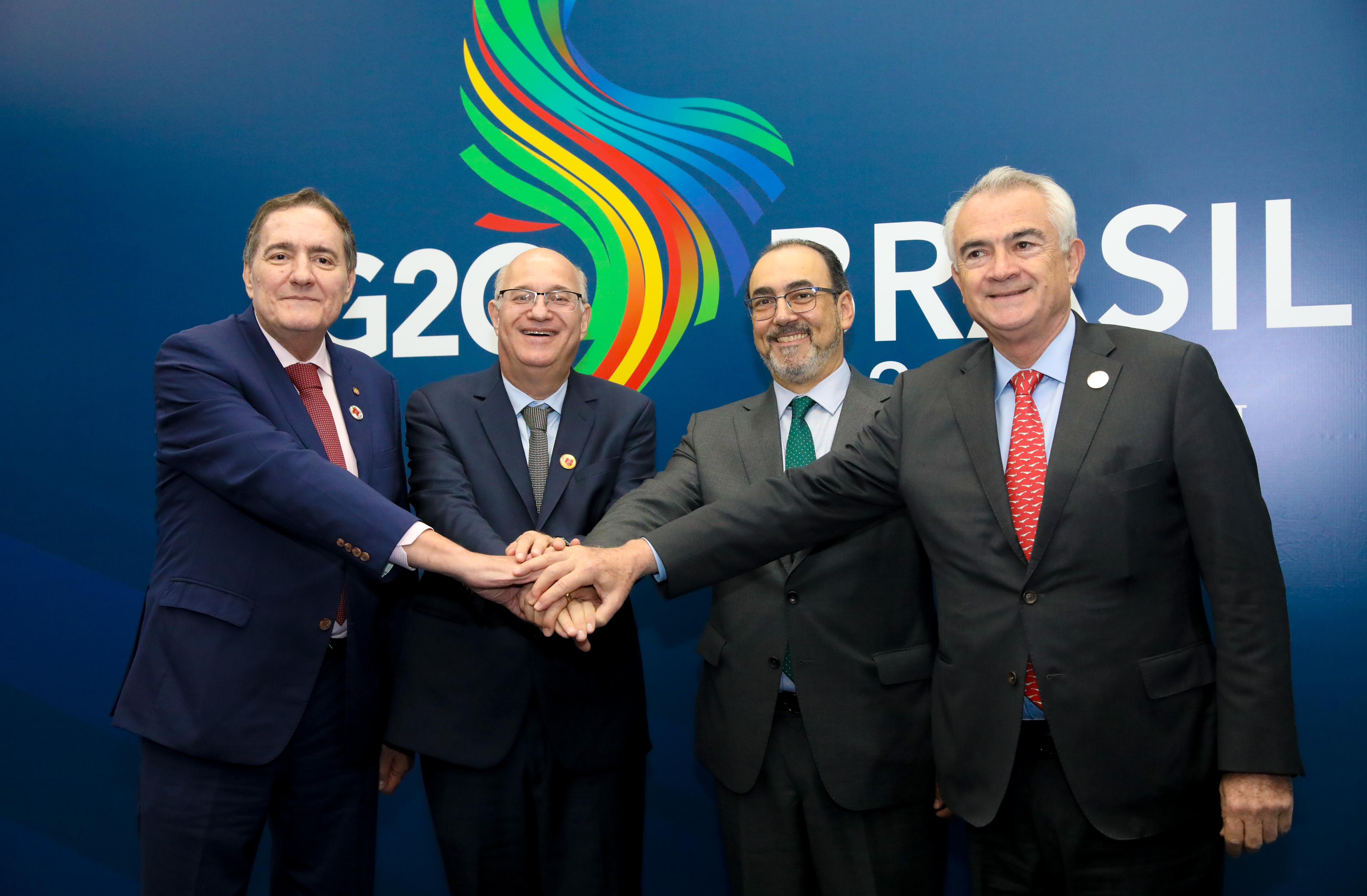
CAF, ECLAC, IDB and PAHO Promote Sustainable Development in the G20
The Regional Organizations of the Americas congratulate Brazil on its successful G20 Presidency, highlighting its leadership on key issues such as poverty, governance, and climate change. They also reaffirm their commitment to actions that promote equity and development in the region.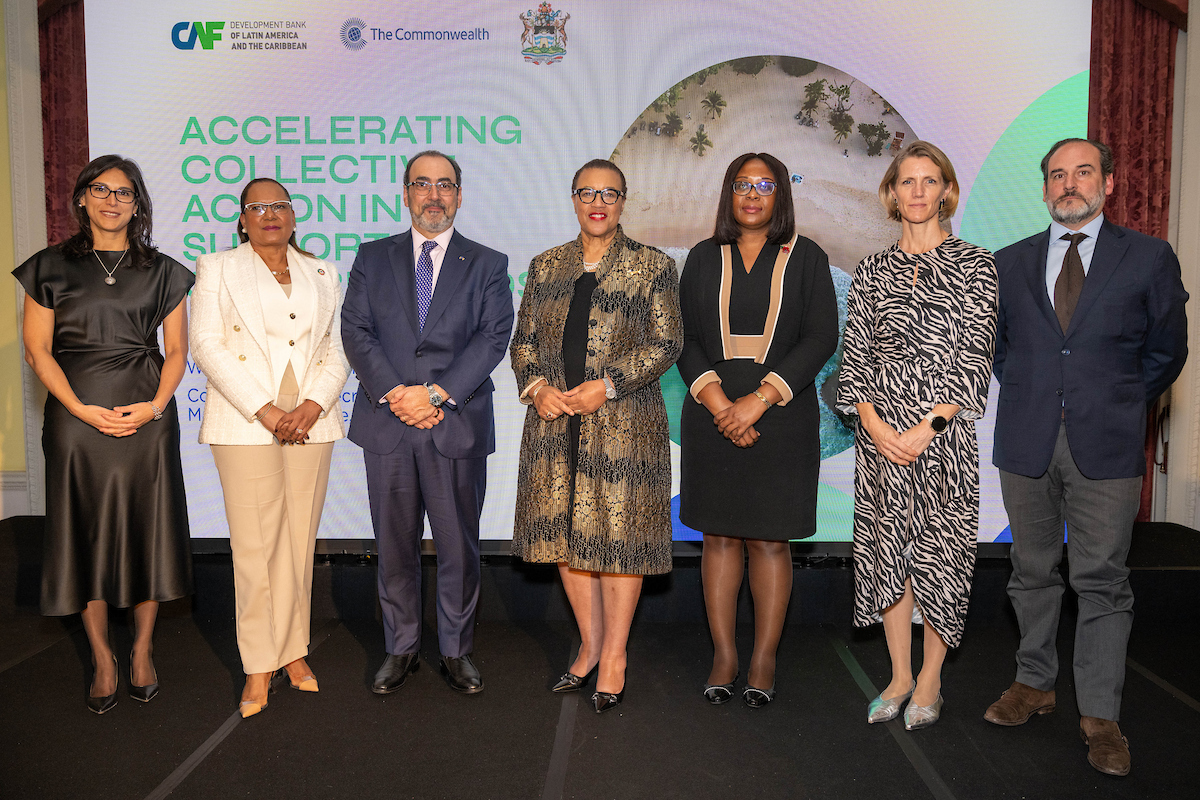
Urgent Call for Action to Safeguard Caribbean SIDS at CAF Symposium
As the global community grapples with the escalating climate crisis, Caribbean Small Island Developing States (SIDS) are running out of time to secure critical investments and support needed to strengthen their economies and protect vulnerable communities from the intensifying impacts of climate change. With the window to take decisive action rapidly shrinking, CAF - Development Bank of Latin America and the Caribbean, in partnership with the Commonwealth Secretariat and the Antigua and Barbuda High Commission, brought together key stakeholders for a symposium in London to address the critical vulnerabilities Caribbean SIDS face.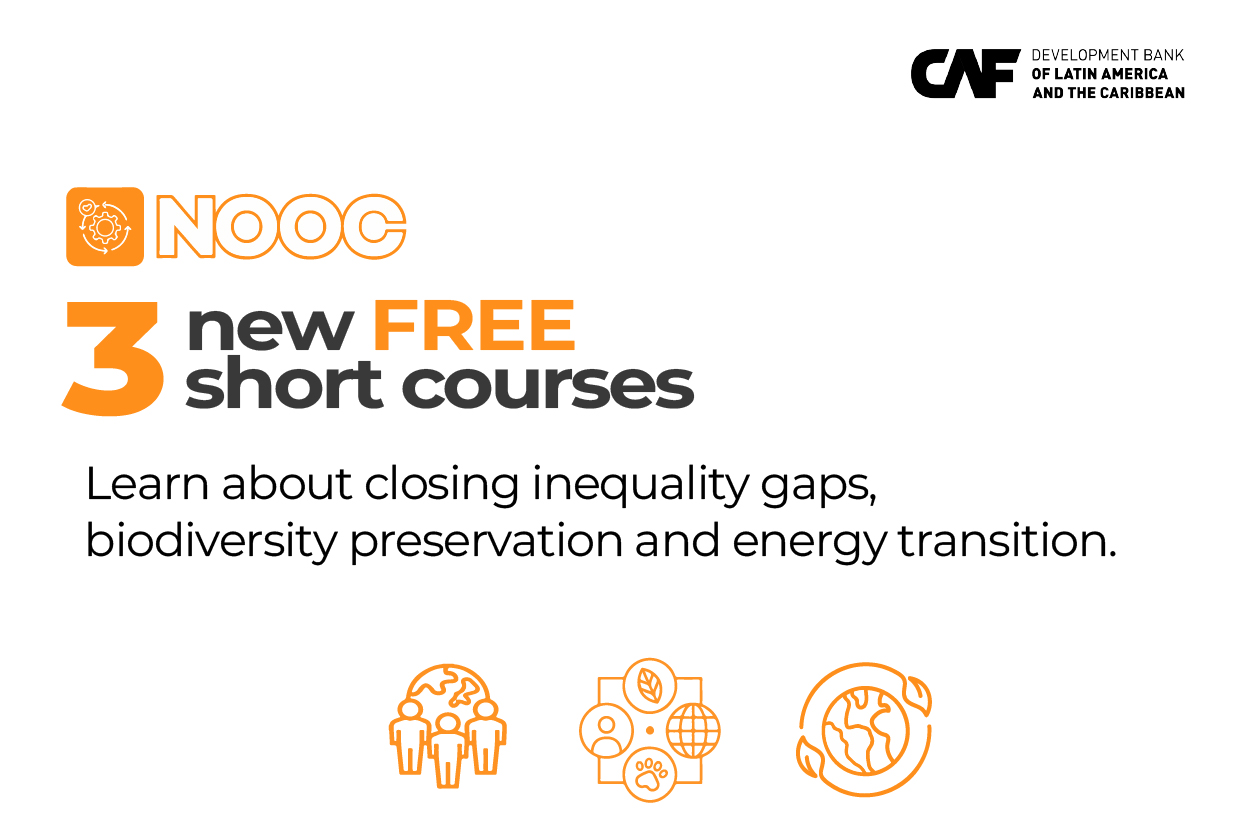