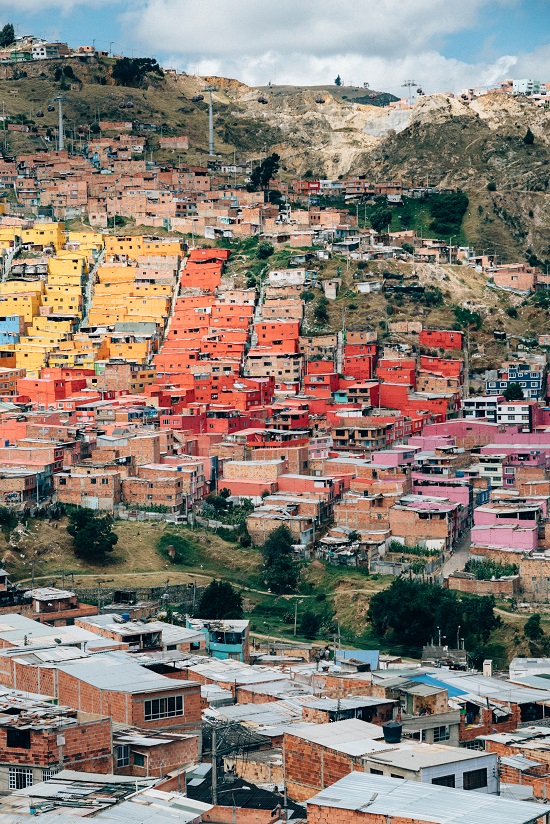
Overcoming Barriers to Randomization in Impact Assessments
One of the challenges we face when designing impact assessments is to randomize program participants to be evaluated. We constantly come across answers such as: “No one can be excluded from the program” or “We have the ability to treat anyone who needs it” or some variation. This means, “We want to assess the impact, but there is no way to do it with an experiment.” An important part of our job is to use creativity to carry out the assessment despite different barriers to randomization.
Some preliminary remarks. It is not our intention to convey the idea that we should always randomize; we are just advising not to rule out so quickly the option to randomize. Secondly, in many cases there are indeed limitations to randomization, but we argue here that they can sometimes be overcome with a little creativity. Third, we believe that considering the alternatives we propose only makes sense if the institution’s need for knowledge is actually related to the impact of a program or an activity.
We could classify hurdles to randomization into “political or ethical” and “logistical” obstacles. “Political or ethical” obstacles are fundamentally a question about the exclusion of a portion of the population in order to assess the impact of a policy. The”logistical” obstacles refer to restrictions imposed by the design of the program to be evaluated: sample size, demand for potential users or program implementation logistics.
Let’s start with the common “No one can be excluded from the program,” which is a “political or ethical” obstacle. The reality is that we usually have to exclude someone, and specific criteria are commonly used for this purpose, especially due to the limitation of resources to serve the entire population. A training program in civil skills for teachers could be implemented only in schools located in post-conflict municipalities in Colombia (almost 16% of municipalities in the country). This targeting criterion excludes by design all teachers who are working in municipalities that are not part of post-conflict areas, and thus, they and their students would be excluded from the potential benefits of training.
In these cases, there is a conscious decision to leave out a portion of the population, and therefore, we must be able to talk openly with our counterparts about fear of exclusion, and explain the fact that there is already a population group that we are excluding based on targeting. Based on this, we could pinpoint the constraint to randomization and how, with targeting criteria, we could find a way to randomize. In our example, the truth is that in addition to targeting post-conflict municipalities, the resources only allow for serving teachers from 80 schools, and thus, we could build a group of at least 200 schools located in post-conflict areas, and choose 80 randomly. If all 200 schools meet the minimum criteria and we can only serve 80 schools, why not select them randomly?
The second obstacle is a variation of the previous hurdle, and is therefore also “political or ethical.” It refers to the fact that sometimes we use criteria based on a belief in the population with the greatest potential benefits to decide who participates in the program: We could implement the teacher training program in the schools most affected by the conflict under the belief (true or not) that the training will be most effective in them. In these circumstances, randomization could mean that schools with a high potential to benefit from the program might end up not receiving it. This raises questions about the benefits of the assessment versus the cost of not benefiting the population that most needs the program.
While this argument is difficult to dispute, we should always ask ourselves in these cases whether we are really sure who needs the program the most (Are we really sure that schools most affected by the conflict are the ones that will benefit most?). These questions compel us to draw on existing evidence and reflect, together with our counterparts, on targeting mechanisms and possible impacts. This reflection can also provide an opportunity to measure the effectiveness of these targeting mechanisms (so we could answer precisely the question of whether the schools that would benefit most from the program are the schools most or least affected).
Other times, the obstacle is that the program has the capacity to serve all those in need. In other words, there is no excess demand, which could be considered as “logistical” obstacle because it is related to program design.
First, if we are narrowing the beneficiary population using an enrollment mechanism through which people may show interest in the program, we must think about how to raise interest in participating in order to generate the excess demand we need to randomize. For example, we could offer the teacher training program in schools whose principals have shown interest in participating. Here, the interest of school principals can increase if we launch a campaign to disseminate the program, which would result in excess demand.
On the other hand, we might also end up with no excess demand. Here, we can try to determine the ability to serve the entire population at the same point in time, as we may need several months (or years) to meet this target. For example, we must reach all 35 districts with training to officials, but with the team of trainers available, we can only serve two districts per month. Thus, in the short term, we do have an excess demand. This can work in our favor when we think about evaluation: We could randomize the order in which distract receive training. In this case of staged treatment, the entire population is treated, but we can assess the impact if we can manage to randomize the order in which treatment occurs for each unit.
Lastly, in some cases it is possible that the implementing institution may have an interest in targeting a specific population, which is not large enough to take up all the available program quota. Here are some additional quotas that can be used to perform the assessment. We want to certify the skills of people employed in the care of the elderly, and the main interest is in people registered in the national healthcare system. It happens that we have the capacity to certify 250 and there are only 100 people registered in the system. This leaves us with a quota of 150 people that we could fill through randomization. The trick is to determine with the institution how many “really need” to receive the program and if there are quotas that are not filled by this population, which could then be used for evaluation.
These are some alternatives that could be used when randomization seems complicated. If no alternative meets the program features we want to evaluate, we might need to consider non-experimental options or wait for the right time to evaluate.
Have you faced any obstacles not mentioned here that you want to share with us? Contact us at impacto@caf.com.
